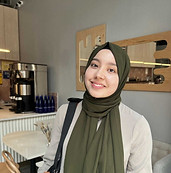
ABOUT ME
I am a research coordinator at Drexel University's Center for Weight, Eating, and Lifestyle Science. My work is focused on understanding the person-specific antecedents of psychopathology, with a particular interest in suicide, non-suicidal self-injury, eating disorders, and substance use.
CONTACT ME
Personal email:
JannahMoussaoui@gmail.com
Institutional email:
jrm486@drexel.edu
LinkedIn:
@JannahMoussaoui
BlueSky:
@JannahMoussaoui.bsky.social
GitHub:
@JannahMoussaoui
Jannah R.
Moussaoui
Personalizing Ecological Momentary Assessment
When designing EMA studies, deciding on when to send surveys is critical. Surveys that come too early or too late risk being missed because the participant is asleep. Similarly, we may need to block certain windows from data sampling due to population-specific considerations. For example, when working with adolescents, we may need to exclude school hours.
In a perfect world, we'd be able to personalize all EMA surveys to participants' schedules, accommodating variations in sleep schedules (e.g., weekend vs. weekday sleep times) or constraints placed by our study protocols. Unfortunately, most EMA platforms do not allow for this level of personalization. In fact, many researchers resort to selecting equidistant time anchors, but this can have an unsightly impact on the distribution of the data (Figure 1A). To remedy this, I built EMAgen, a shiny application that generates personalized EMA schedules which can then be uploaded for delivery, thus yielding more favorable data distributions (Figure 1B).
Figure 1. Simulated distributions (150 participants, 5 surveys a day for 28 days) of EMA surveys under a fixed, equidistant schedule (A) and a person-specific, quasi-random schedule (B). Note that by default, EMAgen over-samples times near wake-up and sleep, hence the bi-modal distribution above.
Metascience
In null hypothesis significance testing (NHST), p-values are evaluated at alpha = 0.05 (conventionally) and used to establish statistical significance for a given effect. There are two principles that apply to p-values (see Figure 2):
-
In the absence of an effect, p-values take on a uniform distribution such that all p-values are equally likely.
-
In the presence of an effect, p-values take on a right-skewed distribution such that smaller p-values are more frequent than larger ones.
Figure 2. Simulated distribution of p-values in the presence of an effect (solid line) and an absent effect (dashed line).
We can take advantage of these principles to estimate the evidential value for a set of statistically significant findings. For instance, p-curve analysis (Simonsohn et al., 2015) compares the distribution of a set of p-values against a uniform distribution. We can also use Bayesian estimation to model a distribution of a set of p-values as a mixture of a uniform and right-skewed distribution (Gronau et al., 2017).
Representative projects:
Moussaoui, J.R., Hyk, A., *Kuhn, K., *Johnson, J., Fischer, S., *Hoxha, B., Munoz Gomez Andrade, F., *Smart-Zimmerman, M., *Do, A., *Gutierrez, B., *Gallik, C., & McCarley, J.S. (2024). P-curving the evidence: P-values published in Human Factors (2017-2023). Proceedings of the Human Factors and Ergonomics Society Annual Meeting.
*Denotes undergraduate mentee
Understanding Heterogeneity
One way we can understand heterogeneity in psychopathology is by identifying subgroups that differ from one another. Finite mixture models, also called latent profile analyses, are parametric probabilistic models that can be used to identify distinct clusters in a dataset. These models are typically used to identify clusters of people, but they can also be used to identify clusters in within-person data (e.g., intra-individual variation in affective or physiological states). Using growth mixture models, we can also identify distinct trajectories in how variables (e.g., symptoms) change over time.